Last week I reviewed at Amazon the terrific new book by Erik Larson titled The Myth of Artificial Intelligence: Why Computers Can’t Think the Way We Do (published by Harvard/Belknap). It is the best available critique of why human intelligence won’t be automated anytime soon. What follows is the review as it appears on Amazon, after which are additional notes that readers of this blog might find interesting.
***
UNSEATING THE INEVITABILITY NARRATIVE
(Five-star Amazon review, posted April 11, 2021)
Back in 1998, I moderated a discussion at which Ray Kurzweil gave listeners a preview of his then forthcoming book THE AGE OF SPIRITUAL MACHINES, in which he described how machines were poised to match and then exceed human cognition, a theme he doubled down on in subsequent books (such as THE SINGULARITY IS NEAR and HOW TO CREATE A MIND). For Kurzweil, it is inevitable that machines will match and then exceed us: Moore’s Law guarantees that machines will attain the needed computational power to simulate our brains, after which the challenge will be for us to keep pace with machines..
Kurzweil’s respondents at the discussion were John Searle, Thomas Ray, and Michael Denton, and they were all to varying degrees critical of his strong AI view. Searle recycled his Chinese Room thought experiment to argue that computers don’t/can’t actually understand anything. Denton made an interesting argument about the complexity and richness of individual neurons and how inadequate is our understanding of them and how even more inadequate our ability is to realistically model them computationally. At the end of the discussion, however, Kurzweil’s overweening confidence in the glowing prospects for strong AI’s future were undiminished. And indeed, they remain undiminished to this day (I last saw Kurzweil at a Seattle tech conference in 2019 — age seemed to have mellowed his person but not his views).
Erik Larson’s THE MYTH OF ARTIFICIAL INTELLIGENCE is far and away the best refutation of Kurzweil’s overpromises, but also of the hype pressed by those who have fallen in love with AI’s latest incarnation, which is the combination of big data with machine learning. Just to be clear, Larson is not a contrarian. He does not have a death wish for AI. He is not trying to sabotage research in the area (if anything, he is trying to extricate AI research from the fantasy land it currently inhabits). In fact, he has been a solid contributor to the field, coming to the problem of strong AI, or artificial general intelligence (AGI) as he prefers to call it, with an open mind about its possibilities.
The problem, as he sees it with the field, is captured in the parable of the drunk looking for keys under a light post even though he dropped them far from it because that’s where the light is. In the spirit of this parable, Larson makes a compelling case that actual research on AI is happening in those areas where the keys to artificial general intelligence simply cannot exist. But he goes the parable even one better: because no theory exists of what it means for a machine to have a cognitive life, he suggests it’s not clear that artificial general intelligence even has a solution — human intelligence may not in the end be reducible to machine intelligence. In consequence, if there are keys to unlocking AGI, we’re looking for them in the wrong places; and it may even be that there are no such keys.
Larson does not argue that artificial general intelligence is impossible but rather that we have no grounds to think it must be so. He is therefore directly challenging the inevitability narrative promoted by people like Ray Kurzweil, Nick Bostrom, and Elon Musk. At the same time, Larson leaves AGI as a live possibility throughout the book, and he seems genuinely curious to hear from anybody who might have some good ideas about how to proceed. His central point, however, is that such good ideas are for now wholly lacking — that research on AI is producing results only when it works on narrow problems and that this research isn’t even scratching the surface of the sorts of problems that need to be resolved in order to create an artificial general intelligence. Larson’s case is devastating, and I use this adjective without exaggeration.
I’ve followed the field of AI for four decades. In fact, I received an NSF graduate fellowship in the early 1980s to make a start at constructing an expert system for doing statistics (my advisor was Leland Wilkinson, founder of SYSTAT, and I even worked for his company in the summer of 1987 — unfortunately, the integration of LISP, the main AI language back then, with the Fortran code that underlay his SYSTAT statistical package proved an intractable problem at the time). I witnessed in real time the shift from rule-based AI (common with expert systems) to the computational intelligence approach to AI (evolutionary computing, fuzzy sets, and neural nets) to what has now become big data and deep/machine learning. I saw the rule-based approach to AI peter out. I saw computational intelligence research, such as conducted by my colleague Robert J. Marks II, produce interesting solutions to well-defined problems, but without pretensions for creating artificial minds that would compete with human minds. And then I saw the machine learning approach take off, with its vast profits for big tech and the resulting hubris to think that technologies created to make money could also recreate the inventors of those technologies.
Larson comes to this project with training as a philosopher and as a programmer, a combination I find refreshing in that his philosophy background makes him reflective and measured as he considers the inflated claims made for artificial general intelligence (such as the shameless promise, continually made, that it is just right around the corner — is there any difference with the Watchtower Society and its repeated failed prophecies about the Second Coming?). I also find it refreshing that Larson has a humanistic and literary bent, which means he’s not going to set the bar artificially low for what can constitute an artificial general intelligence.
The mathematician George Polya used to quip that if you can’t solve a given problem, find an easier problem that you can solve. This can be sound advice if the easier problem that you can solve meaningfully illuminates the more difficult problem (ideally, by actually helping you solve the more difficult problem). But Larson finds that this advice is increasingly used by the AI community to substitute simple problems for the really hard problems facing artificial general intelligence, thereby evading the hard work that needs to be done to make genuine progress. So, for Larson, world-class chess, Go, and Jeopardy playing programs are impressive as far as they go, but they prove nothing about whether computers can be made to achieve AGI.
Larson presents two main arguments for why we should not think that we’re anywhere close to solving the problem of AGI. His first argument centers on the nature of inference, his second on the nature of human language. With regard to inference, he shows that a form of reasoning known as abductive inference, or inference to the best explanation, is for now without any adequate computational representation or implementation. To be sure, computer scientists are aware of their need to corral abductive inference if they are to succeed in producing an artificial general intelligence. True, they’ve made some stabs at it, but those stabs come from forming a hybrid of deductive and inductive inference. Yet as Larson shows, the problem is that neither deduction, nor induction, nor their combination are adequate to reconstruct abduction. Abductive inference requires identifying hypotheses that explain certain facts of states of affairs in need of explanation. The problem with such hypothetical or conjectural reasoning is that that range of hypotheses is virtually infinite. Human intelligence can, somehow, sift through these hypotheses and identify those that are relevant. Larson’s point, and one he convincingly establishes, is that we don’t have a clue how to do this computationally.
His other argument for why an artificial general intelligence is nowhere near lift-off concerns human language. Our ability to use human language is only in part a matter of syntactics (how letters and words may be fit together). It also depends on semantics (what the words mean, not only individually, but also in context, and how words may change meaning depending on context) as well as on pragmatics (what the intent of the speaker is in influencing the hearer by the use of language). Larson argues that we have, for now, no way to computationally represent the knowledge on which the semantics and pragmatics of language depend. As a consequence, linguistic puzzles that are easily understood by humans and which were identified over fifty years ago as beyond the comprehension of computers are still beyond their power of comprehension. Thus, for instance, single-sentence Winograd schemas, in which a pronoun could refer to one of two antecedents, and where the right antecedent is easily identified by humans, remains to this day opaque to machines — machines do no better than chance in guessing the right antecedents. That’s one reason Siri and Alexa are such poor conversation partners.
THE MYTH OF ARTIFICIAL INTELLIGENCE is not just insightful and timely, but it is also funny. Larson, with an insider’s knowledge, describes how the sausage of AI is made, and it’s not pretty — it can even be ridiculous. Larson retells with enjoyable irony the story of Eugene Goostman, the Ukranian 13-year old chatbot, who/which through sarcasm and misdirection convinced a third of judges in a Turing test, over a five-minute interaction, that it was an actual human being. No, argues Larson, Goostman did not legitimately pass the Turing test and computers are still nowhere near passing it, especially if people and computers need to answer rather than evade questions. With mirth, Larson also retells the story of Tay, the Microsoft chatbot that very quickly learned how to make racist tweets, and got him/itself just as quickly retired.
And then there’s my favorite, Larson’s retelling of the Google image recognizer that identified a human as a gorilla. By itself that would not be funny, but what is funny is what Google did to resolve the problem. You’d think that the way to solve this problem, especially for a tech giant like Google, would be simply to fix the problem by making the image recognizer more powerful in its ability to discriminate humans from gorillas. But not Google. Instead, Google simply removed all references to gorillas from the image recognizer. Problem solved! It’s like going to a doctor with an infected finger. You’d like the doctor to treat the infection and restore the finger to full use. But what Google did is more like a doctor just chopping off your finger. Gone is the infection. But — gosh isn’t it too bad — so is the finger.
We live in a cultural climate that loves machines and where the promise of artificial general intelligence assumes, at least for some, religious proportions. The thought that we can upload ourselves onto machines intrigues many. So why not look forward to the prospect of them doing so, especially since some very smart people guarantee that machine supremacy is inevitable. Larson in THE MYTH OF ARTIFICIAL INTELLIGENCE successfully unseats this inevitability narrative. After reading this book, believe if you like that the singularity is right around the corner, that humans will soon be pets of machines, that benign or malevolent machine overlords are about to become our masters. But after reading this book, know that such a belief is unsubstantiated and that neither science nor philosophy backs it up.
ADDITIONAL NOTES
There’s a lot more I would have liked to say in this review, but I didn’t want the review to get too long and I also wanted to get it done. Here are some additional notes (to which I may add over time):
NOTE 1. Three of the failures of big tech listed above (Eugene Goostman, Tay, and the image analyzer that Google lobotomized so that it could no longer detect gorillas, even mistakenly) were obvious frauds and/or blunders. Goostman was a fraud out of the box. Tay a blunder that might be fixed in the sense that its racist language could be mitigated through some appropriate machine learning. And the Google image analyzer — well that was just pathetic: either retire the image analyzer entirely or fix it so that it doesn’t confuse humans with gorillas. And if you can’t fix it, so much the worse for AI’s grand pretensions. Such failures suggest that an imminent machine takeover is unlikely. But there are more systemic failures, to which Larson draws our attention. I’ll take some of these up in the next notes.
NOTE 2. The failure of automated driving to reach level 5 (i.e., full automation with no need whatsoever of human guidance) remains not just out of reach, but seems to be getting more out of reach even as machine/deep learning gets more powerful and sophisticated. This itself suggests that the approach being taken is ill-starred given that both software and hardware are, as a matter of technological progress, getting better, and yet they are yielding diminishing, and now it appear vanishing, returns in the automation of driving. Larson makes the point more starkly in his book: “Self-driving cars are an obvious case in point [the point being that, as Larson writes earlier, these “systems are idiots”]. It’s all well and good to talk up advances in visual object recognition until, somewhere out on the long tail of unanticipated consequences and therefore not included in the training data, your vehicle happily rams a passenger bus as it takes care to miss a pylon. (This happened.)” I’ve made similar arguments on this blog here and here.
NOTE 3. Taking the challenge of developing fully automated driving one step further, it would be interesting to see what fully automated driving would look like in a place like Moldova. A US friend of mine who happened to visit the country was surprised at how Moldovan drivers managed to miss hitting each other despite a lack of clear signals and rules about when to take an opportunity and when to hold back. When he asked his Moldovan guide how the drivers managed to avoid accidents, the guide answered with two words: “eye contact.” Apparently, the drivers could see in each other’s eyes who was willing to hold back and who was ready to move ahead. Now that’s a happy prospect for fully automated driving. Perhaps we need “level 6” automation, in which AI systems have learned to read the eyes of drivers to determine whether they going are to restrain themselves or make that left turn into oncoming traffic. This example suggests to me that AI is hopelessly behind the full range of human cognitive capabilities. It also suggests that we, in the cossetted and sanitized environments that we have constructed for ourselves in the US, have no clue of what capabilities AI actually needs to achieve to truly match what humans can do (the shortfall facing AI is extreme).
NOTE 4. With natural language processing, Larson amusingly retells the story of Joseph Weizenbaum’s ELIZA program, in which the program, acting as a Rogerian therapist, simply mirrors back to the human what the human says. Carl Rogers, the psychologist, advocated a “non-directive” form of therapy where, rather than tell the patient what to do, the therapist reflected back what the patient was saying, as a way of getting the patient to solve one’s own problems. Much like Eugene Goostman above, ELIZA is a cheat, though to its inventor Weizenbaum’s credit, he recognized from the get-go that it was a cheat. ELIZA was a poor man’s version for passing the Turing test, taking in text from a human and regurgitating it in a modified form. To the statement “I’m lonely today,” the program might respond “Why are you lonely today?” All that’s required of the program here is some simple grammatical transformations, changing a declarative statement into a question, a first person pronoun to a second person pronoun, etc. No actual knowledge of the world or semantics is needed.
In 1982, I sat in on an AI course at the University of Illinois at Chicago where the instructor, a well-known figure in AI at the time by name of Laurent Siklossy, gave us, as a first assignment, to write an ELIZA program. Siklossy, a French-Hungarian, was a funny guy. He had written a book titled Let’s Talk LISP (that’s funny, no?), LISP being the key AI programming language at the time and the one in which we were to write our version of ELIZA. I still remember the advice he gave us in writing the program:
- We needed to encode a bunch of grammatical patterns so that we could turn around sentences written by a human in a way that would reflect back what the human wrote, whether as a question or statement.
- We needed to make a note of words like “always” or “never,” which were typically too strong and emotive, and thus easy places to ask for elaboration (e.g., HUMAN: “I always mess up my relationships.” ELIZA: “Do you really mean ‘always’? Are there no exceptions?”).
- And finally — and this remains the funniest thing I’ve ever read or heard in connection with AI — he advised that if a statement by a human to the ELIZA program matched no grammatical patterns or contained no salient words that we had folded into our program (in other words, our program drew a blank), we should simply have the program respond, “I understand.”
That last piece of advice by Siklossy captures for me the essence of AI — it understands by not understanding!
NOTE 5. “Deep learning” is as misnamed a computational technique as exists. The actual technique refers to multi-layered neural networks, and, true enough, those multi-layers can do a lot of significant computational work. But the phrase “deep learning” suggests that the machine is doing something profound and beyond the capacity of humans. That’s far from the case. The Wikipedia article on deep learning is instructive in this regard. Consider the following image used there to illustrate deep learning:
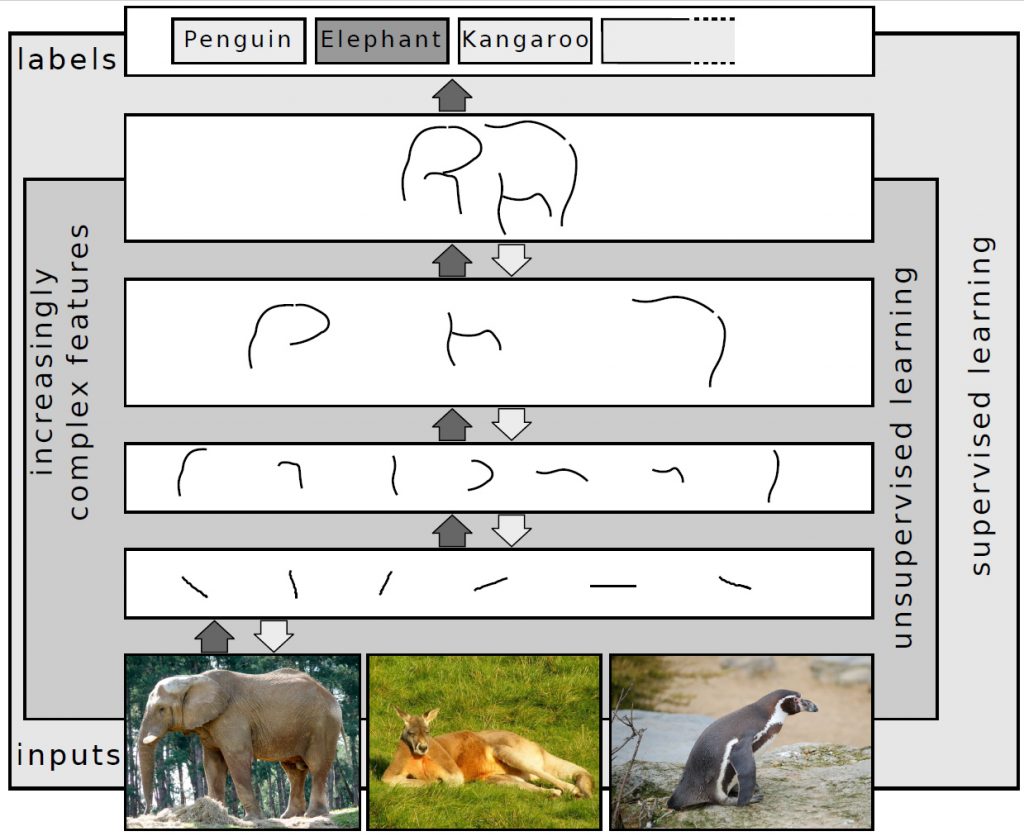
Note the rendition of the elephant at the top and compare it with the image of the elephant as we experience it at the bottom. The image at the bottom is rich, textured, colorful, and even satisfying. What deep learning extracts, and what is rendered at the top, is paltry, simplistic, black-and-white, and unsatisfying. What’s at the top is what deep learning “understands” — in fact, its “understanding,” whatever we might mean by the term, cannot progress beyond what is rendered at the top level. This is pathetic, and this is what is supposed to lay waste and supersede human intelligence? Really now.
NOTE 6. The event at which I moderated the discussion about Ray Kurzweil’s THE AGE OF SPIRITUAL MACHINES was the 1998 George Gilder TELECOSM conference, which occurred in the fall of that year at Lake Tahoe (I remember baseball players Sammy Sosa and Mark McGwire chasing each other for home run leadership at the time). In response to the discussion, I wrote a paper for FIRST THINGS titled “Are We Spiritual Machines?” — it is still available online at the link just given, and its arguments remain current and relevant. An abridged version of this article then appeared in an anthology, edited by my friend and colleague Jay Richards: ARE WE SPIRITUAL MACHINES? RAY KURZWEIL AND THE CRITICS OF STRONG AI. This anthology is still available and still worth attention, if only to point out how the same themes and misconceptions about AI persist.
NOTE 7. Erik Larson did an interesting podcast with the Brookings Institution through its Lawfare Blog shortly after the release of his book. It’s well worth a listen, and Larson elucidates in that interview many of the key points in his book. The one place in the interview where I wish he had elaborated further was on the question of abductive inference (AKA retroductive inference or inference to the best explanation). For me, the key to understanding why computers cannot, and most likely will never, be able to perform abductive inferences is the problem of underdetermination of explanation by data. This may seem like a mouthful, but the idea is straightforward. For context, if you are going to get a computer to achieve anything like understanding in some subject area, it needs a lot of knowledge. That knowledge, in all the cases we know, needs to be painstakingly programmed. This is true even of machine learning situations where the underlying knowledge framework needs to be explicitly programmed (for instance, even Go programs that achieve world class playing status need many rules and heuristics explicitly programmed).
Humans, on the other hand, need none of this. On the basis of very limited or incomplete data, we nonetheless come to the right conclusion about many things (yes, we are fallible, but the miracle is that we are right so often). Noam Chomsky’s entire claim to fame in linguistics really amounts to exploring this underdetermination problem, which he referred to as “the poverty of the stimulus.” Humans pick up language despite very varied experiences with other human language speakers. Babies born in abusive and sensory deprived environments pick up language. Babies subject to Mozart from the womb and with rich sensory environments pick up language. Language results from growing up with cultured and articulate parents. Language results from growing up with boorish and inarticulate parents. Yet in all case, the actual amount of language exposure is minimal compared to language ability that emerges and the knowledge of the world that results. On the basis of the language exposure, many different ways of understanding the world might have developed, and yet we seem to get things right (much of the time). Harvard philosopher Willard Quine, in his classic WORD AND OBJECT (1960), struggled with this phenomenon, arguing for what he called the indeterminacy of translation to make sense of it.
The problem of underdetermination of explanation by data appears not just in language acquisition but in abductive inference as well. It’s a deep fact of mathematical logic (i.e., the Löwenheim–Skolem theorem) that any consistent collection of statements (think data) has infinitely many mathematical models (think explanations). This fact is reflected in ordinary everyday abductive inferences. We are confronted with certain data, such as missing documents from a bank safety deposit box. There are many many ways this might be explained: a thermodynamic accident in which the documents spontaneously combusted and disappeared, a corrupt bank official who stole the documents, a nefarious relative who got access and stole the documents, etc.
But the “et cetera” here has no end. Maybe it was space aliens. Maybe you yourself took and hid the documents, and are now suffering amnesia. There are a virtually infinite number of possible explanations. And yet, somehow, we are often able to determine the best explanation, perhaps with the addition of more data/evidence. But even adding more data/evidence doesn’t eliminate the problem because however much data/evidence you add, the underdetermination problem remains. You may eliminate some hypotheses (perhaps the hypothesis that the bank official did it, but not other hypotheses). But by adding more data/evidence, you’ll also invite new hypotheses. And how do you know which hypotheses are even in the right ballpark, i.e., that they’re relevant? Why is the hypothesis that the bank official took the documents more relevant than the hypothesis that the local ice cream vendor took them? What about the local zoo keeper? We have no clue how to program relevance, and a fortiori we have no clue how to program abductive inference (which depends on assessing relevance). Larson makes this point brilliantly in his book.
NOTE 8. Here’s a terrific video interview that Erik Larson did with Academic Influence. It was done before his book was released and gives a succinct summary of the book. It’s short (15 minutes, compared to the hour-long interview with Brookings described in the previous note).
For not only the full video of this interview with Larson but also a transcript of it, go to the Academic Influence website here.
NOTE 9. For a nice period-piece video on Weizenbaum’s ELIZA program, check out this YouTube video: